In today’s rapidly evolving product landscape, product managers face the daunting task of making strategic decisions that drive both business and customer success. Despite their best efforts, many still grapple with a fundamental challenge: the lack of sufficient data to confidently guide investment decisions. According to the ProductPlan State of Product Management Report 2024, product strategy remains the most critical aspect of a product manager’s role, yet its effectiveness hinges on the availability of robust tools and frameworks for measuring success. The same report also highlights a significant shift in focus—product managers are increasingly moving away from maximizing outputs and instead striving to drive sustained user value through the satisfaction of outcomes.
This growing emphasis on outcomes reflects a broader industry trend. As predicted in recent product management forecasts, there is a clear movement towards distinguishing superficial innovations from those that genuinely enhance the user experience. This evolution in product development signals a shift from merely focusing on outputs to emphasizing impact. The real challenge for product managers, however, lies in adopting new frameworks to understand and articulate what true impact looks like, and more importantly, in finding ways to measure it effectively. This gap underscores the need for more sophisticated tools that can bridge the divide between user behaviors and business objectives, ensuring that features not only perform well but also create meaningful, lasting impact.
In a previous article, I introduced the User Outcome Connection, a framework designed to define the intended impact of each feature. This framework consists of three primary components: the user outcome, which explains why a person engages with the feature; specific behaviors that, when altered, lead to the desired outcome; and the business impact that is achieved when the outcome is fulfilled. Together, these components form the basis of a measurable approach to defining a feature’s purpose and evaluating its success.
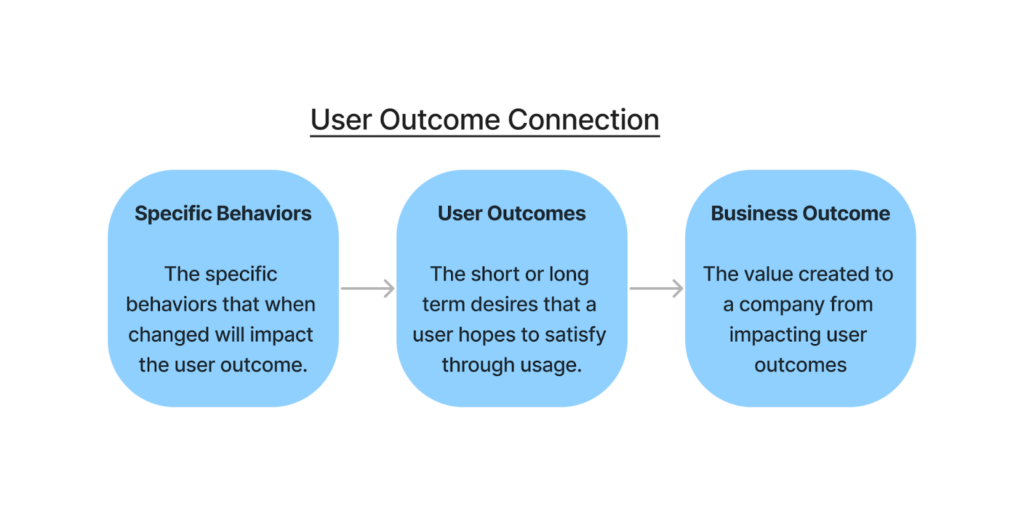
Formalizing the process of defining features allows for a structured approach to measurement, enabling teams to validate whether their features are actually achieving their intended goals. This structured approach not only supports evidence-based investment decisions but also facilitates the transfer of learnings across features. Filling out the User Outcome Connection is the first step in a more comprehensive process to validate these connections with data. This process, which I detail in my recently published book, Bridging Intentions to Impact, is known as the feature impact analysis.
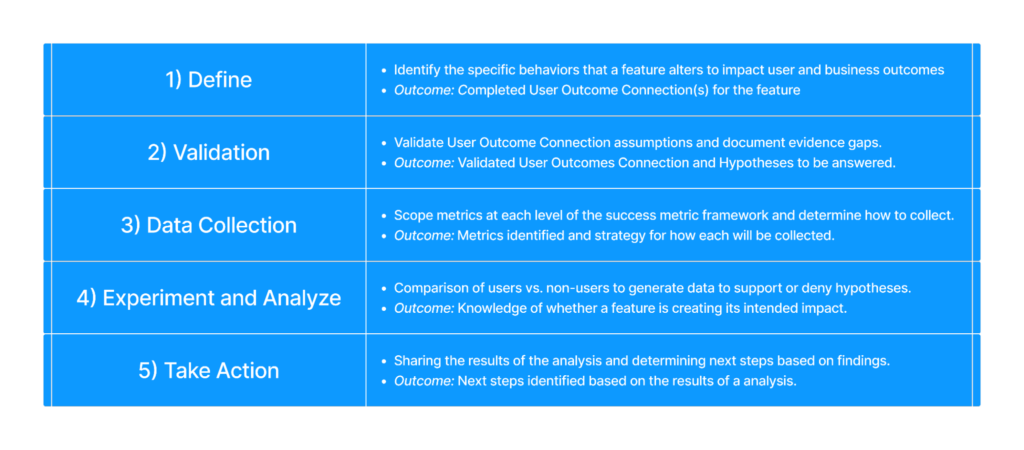
Starting a feature impact analysis begins with the Define phase, where the focus is on establishing the User Outcome Connection. This involves identifying the specific behaviors that a feature is intended to influence, the user outcomes these behaviors are meant to achieve, and the corresponding business outcomes that will result. The process of defining a feature can vary depending on its origins—whether it was born from direct research findings or from a small group’s strong product intuition. For some features, this may be straightforward, while others, with more marginal reasons for existing, may require a more structured approach. This phase ensures that everyone involved has a shared understanding of what the feature is supposed to accomplish, setting the stage for effective measurement and analysis.
After defining the connections between behaviors, user outcomes, and business outcomes, the next phase is Validate. Here, the team tests the assumptions made during the Define phase by examining existing data to determine whether it supports the expected connections. This involves validating three primary assumptions: whether the feature effectively changes the behavior, whether altering the behavior leads to the desired outcomes, and whether achieving those outcomes results in a measurable business impact. Often, this process reveals gaps in the data—areas where the relationships between behaviors and outcomes are not fully supported by evidence. Identifying these gaps is crucial, as it highlights where additional data collection or further analysis is needed to accurately assess the feature’s impact.
The third step, the Data Collection phase, addresses the gaps identified in the Validate step by establishing the metrics needed to measure the connections within the User Outcome Connection framework. Conducted in two parts, this starts with the functional design of the metric, determining what exactly would be needed to measure each component. The second part is actually implementing new data collection methods to capture the missing information. Here, trade-offs may be required, and teams should be comfortable with using proxy metrics if they represent an improvement over existing data. Whether it involves developing new tracking mechanisms, gathering user feedback, or setting up experiments, this step ensures that all necessary data is available for a comprehensive analysis of the feature’s impact. The metrics collected during this phase lay the groundwork for evaluating the feature’s effectiveness in the subsequent steps.
With the necessary data in hand, the Experiment and Analyze phase begins. In this step, product teams conduct experiments to compare the experiences of users who engage with the feature against those who do not. The goal is to determine whether the feature is truly driving the intended behavioral changes and achieving the desired outcomes. By analyzing the results, teams can quantify the feature’s impact, validating or refuting the initial hypotheses. This phase is critical for understanding the real-world effectiveness of a feature and for making informed decisions about its future.
Experimentation can often seem daunting, leading some teams to shy away from it. However, it’s important to recognize that there are many types of experiments, and almost any organization has the means to start with something small. Not every test needs to meet the rigorous standards of academic research; even basic experiments can yield insights that are better than relying on intuition alone. Similarly, while advanced statistical analyses can be beneficial, sometimes basic descriptive statistics are all that’s needed to guide the team toward a valuable outcome.
The final step in the Feature Impact Analysis is the Take Action phase, where the insights gained from the previous phases are put into practice. Depending on the results, this could mean optimizing the feature to enhance its impact, scaling it to reach more users, or even retiring it if it fails to deliver the expected outcomes. Additionally, the findings are communicated to stakeholders, ensuring that the evidence-based insights inform broader product strategies and decisions. This step not only drives immediate improvements but also contributes to building a culture of continuous learning and data-driven decision-making within the organization.
By following these FIA phases, product teams not only gain a solid, evidence-backed definition of each feature but also establish new measurements that provide clarity on their effectiveness. This approach turns assumptions into actionable data, offering valuable insights into what is truly working and what isn't. As a result, teams can confidently invest in features, knowing they are making informed decisions based on real impact rather than intuition. Ultimately, FIA empowers organizations to drive meaningful outcomes, ensuring that every feature contributes to the product's success and aligns with broader business goals.
The journey of creating a FIA begins by building your own User Outcome Connection, a foundational exercise that will help you understand the intended impact of each feature. Start small—select a feature that is manageable in scope and significant enough to demonstrate value. This initial step allows you to clearly outline the behaviors you aim to influence, the outcomes you hope to achieve, and the business impact you expect. By completing this exercise, you’ll gain a clearer perspective on what success looks like for that feature, setting the stage for the more detailed analysis that follows.
Once you’ve established the User Outcome Connection, the next challenge is validating these connections with data. This is where FIA truly comes to life. Begin by gathering existing data to test your assumptions, and identify where gaps might exist. If certain connections lack sufficient evidence, consider how you can collect the necessary data—whether through new metrics, user feedback, or simple experiments. Remember, the goal is not to create a perfect system overnight but to start refining your approach to measurement and impact evaluation. The insights you gain from this process will guide your decisions, ensuring that your product development is based on evidence rather than intuition alone.
Finally, it’s crucial to recognize that FIA is more than just a tool for analyzing individual features—it’s a step towards fostering a culture of evidence-based decision-making within your organization. As you gather data and validate your feature impacts, share your findings with your team and stakeholders. Use these insights to drive conversations about product strategy and investment, and encourage others to adopt a similar approach. Over time, this practice will help embed a mindset focused on impact, leading to more strategic, data-driven product development across your organization.
For those who are ready to dive deeper into the process, my book “Bridging Intention to Impact” offers a comprehensive guide to FIA and its applications. It covers everything from the initial steps of defining and validating features to advanced techniques for data collection and analysis. Whether you’re new to this approach or looking to refine your existing processes, the book provides the tools and insights needed to ensure that every feature you build creates the impact you intend. By embracing FIA, you’ll be better equipped to make informed decisions, drive meaningful outcomes, and ultimately, deliver products that truly succeed.
Connor is a keynote speaker at #mtpcon North America this October in Raleigh, NC. Connor's keynote is titled 'AI Features Demand Evidence-Based Decisions'.
Don't miss out on this opportunity to learn from product leaders - buy your ticket!
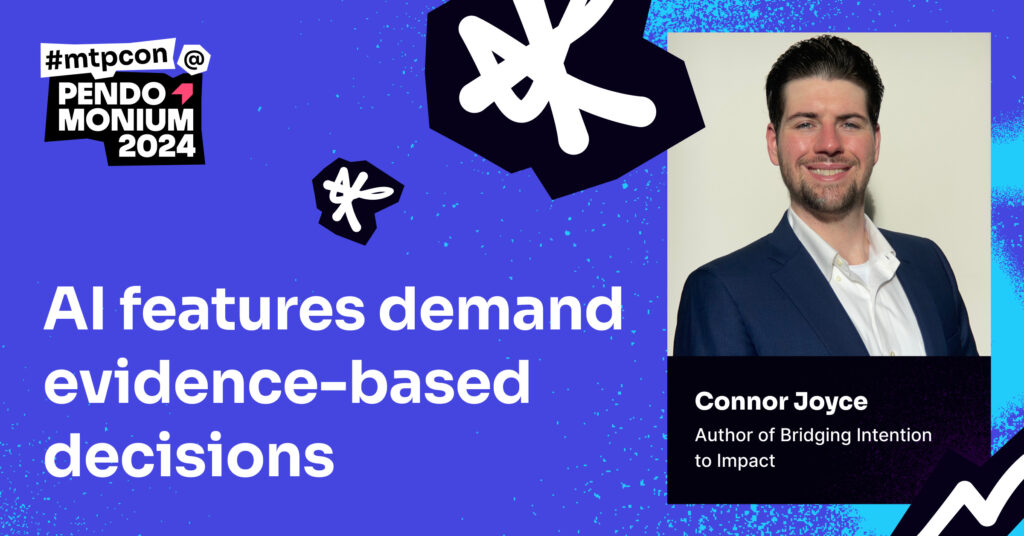
Comments
Join the community
Sign up for free to share your thoughts